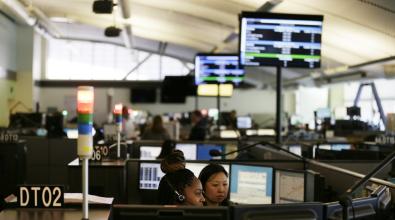
Taking AI experimentation to the next level in cities
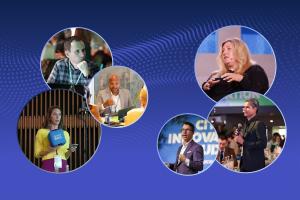
Photos courtesy of Bloomberg Philanthropies
Listen to This Article
From fast-tracking the procurement process to guiding residents in accessing critical services to engaging citizens in long-term planning, generative artificial intelligence is beginning to change how cities work. But it's not the technology, alone, that is driving these changes—it’s also local leaders working to identify the most appropriate uses for the technology. And at Bloomberg Philanthropies, we believe that it is by consistently experimenting with how generative AI can be integrated into their daily practices that city innovators will harness its full potential while being mindful of its limitations.
To deepen their understanding of generative AI and how it can be ethically applied to their work, 120 data and innovation leaders from over 90 cities recently came together for a City Innovation Studio at Bloomberg CityLab in Mexico City. There, they dug into exactly how their innovation skills can take AI experimentation to the next level—and what this work can, in turn, produce for the people they serve.
Introducing the “jagged frontier” of AI in government.
Mitchell Weiss, a Harvard Business School professor and senior advisor to the Bloomberg Harvard City Leadership Initiative, introduced those gathered in Mexico City to an overarching framework for being more ambitious with artificial intelligence in their work. The approach builds off of a working paper co-authored by some of his colleagues, which stipulates that genAI helps people increase speed and produce higher-quality results when it’s used to tackle specific tasks within the “jagged frontier.” This frontier is best understood as a curve delineating what falls “inside” and “outside” the technology’s current set of capabilities.
While the paper was exploring the use of genAI in the private sector, Weiss believes embracing the idea of a jagged frontier can help city innovators do more with the technology on behalf of the public. What he means in practice is that they won’t know which tasks fall inside and outside generative AI’s capabilities until they find out where that jagged frontier falls for themselves. That means pursuing constant, bold experimentation, which is exactly what they did as a group in Mexico City.
“The thing about AI, at least generative AI, is you don't actually know what it will do,” Weiss tells Bloomberg Cities. “There's no actual user manual. There's no finite list of tasks it can perform, either with you or at your direction, until you try.”
Experimenting to reveal usefulness and grapple with limitations.
The specific tasks that fall on either side of the jagged frontier—that are or are not within generative AI’s current capabilities—may prove surprising to city leaders who have not yet spent considerable time with the technology. As Weiss demonstrated at the City Innovation Studio, a request as simple as producing a group of sentences that are each a dozen words long was too difficult for one of the most popular of these AI tools.
“The tools are not built to be quantitative tools. They're language models,” Weiss explains, before noting that what works one week or one month may be different the next: “They're getting more and more quantitative capacity as we go.”
Weiss believes that if anything, city leaders may be at risk of not fully appreciating just how useful this technology can already be right now. At the City Innovation Studio, local innovators gained perspective on that when they took on a hypothetical challenge to prototype a new solution for heat mitigation in a city—and found that generative AI tools produced a wide range of concrete deliverables in virtually no time at all. Specifically, generative AI helped the innovators very quickly identify useful data sources, generate fresh ideas, consider case studies from other cities, and create minimal viable product (MVP) templates and project documentation.
“They used the tools for problem definition, for ideation, and for prototyping, and in a very short period of time—it was a real kind of hair-on-fire exercise—they came up with really amazing outcomes,” says Francisca Rojas, academic director of the Bloomberg Center for Public Innovation at Johns Hopkins University.
Improving data and other key inputs to produce better results.
Just as city innovators know it’s always important that they test and evaluate and adjust solutions as necessary, it can be helpful for them to keep an open mind that a task falling outside of AI’s current capabilities isn’t necessarily a reason to stop trying. In other words, past behavior is not a predictor of future capacity when it comes to generative AI tools.
One example that surfaced at the session of a task potentially being outside the frontier—understanding gaps in services for a specific demographic group in a specific city—represented an opportunity to improve the tool, rather than a failure, Weiss noted.
“One question you should have in your mind with these things that we think are outside the frontier [is] whether you could, somehow, by virtue of your skill or knowledge basis, bring these things actually into the frontier,” he told city leaders. After all, in a case like this, a city may simply not have published clear documentation of its limited capacity for service delivery. To incorporate that in its work, a generative AI tool needs to be trained on the relevant dataset.
Likewise, a task such as grasping the perspective of a minority community living in a city might, initially, appear to be beyond the technology’s capabilities. Certainly, these tools cannot replace engagement with actual residents. But a core learning here is that if leaders point generative AI tools to new data and new perspectives, they can begin to address these shortcomings.
“What we're seeing is that the cities that are actively engaged in finding ways that this kind of general-purpose technology can be adopted and integrated into city operations [are] training the models with their own data,” Rojas explains.
At the same time, Beth Blauer—associate vice provost for public sector innovation at Johns Hopkins University, where she oversees the Bloomberg Center for Government Excellence and the Bloomberg Center for Public Innovation—advises that cities bear in mind that generative AI doesn't, on its own, include every precaution that needs to be a part of every government activity.
“It wasn't designed to protect personally identifying information. It wasn't designed to take into account the regulatory frameworks that government is up against when it has to navigate decisions,” she notes. Even so, she encourages cities to explore the jagged frontier “to understand what it’s good at, what it's not good at, and then demand” more from their partners in the private sector that are developing these tools.
So cities will always need to take care to use engagement to bring residents along for the ride and be mindful of ethical considerations with their genAI work. If they do so, city innovators are now positioned to lead the entire public sector forward.
“Use it on what you do every day,” Weiss says. “I know that doesn't sound profound, but that's what I would advise.”